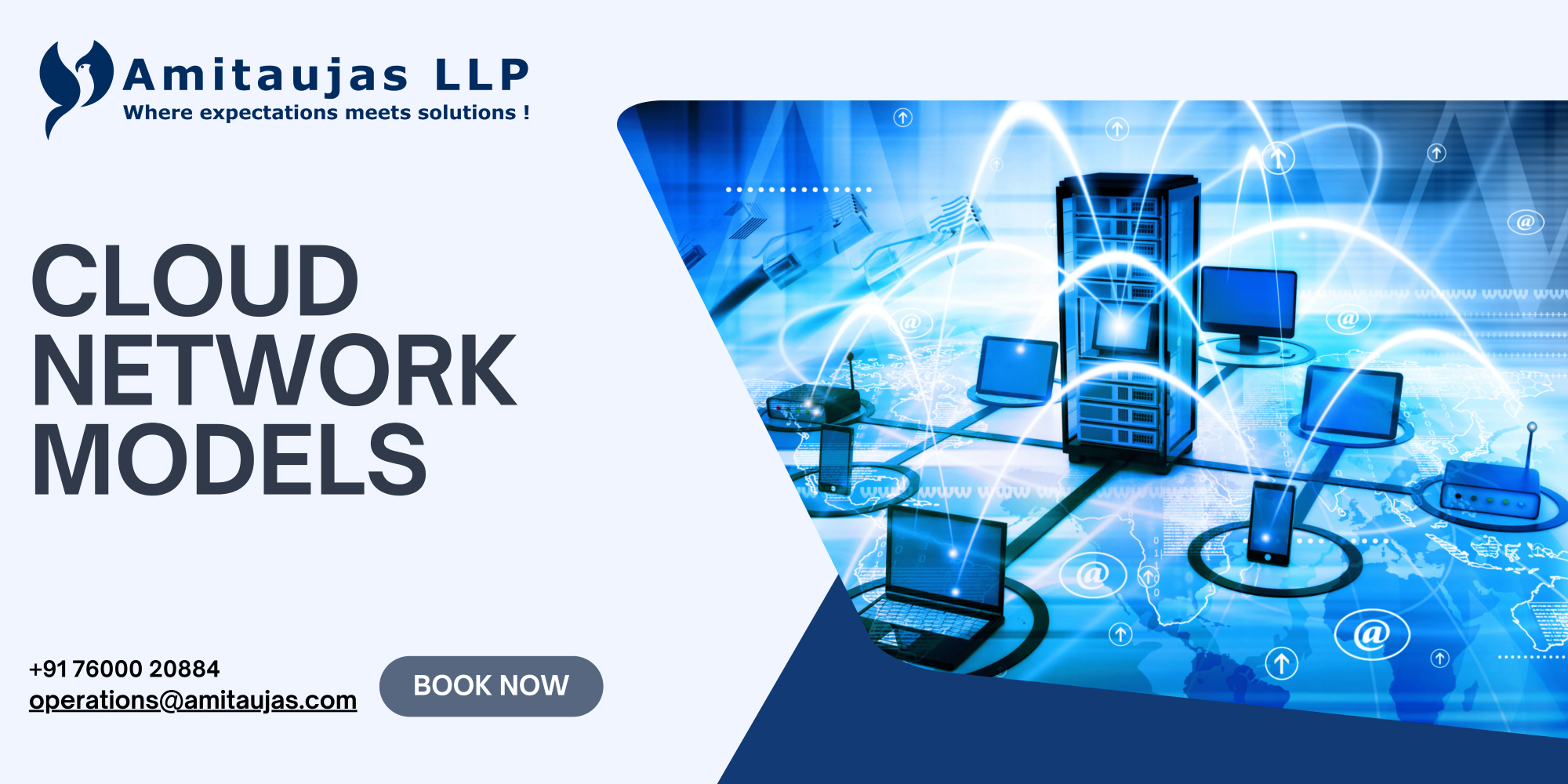
Cloud Network Models
1. Cloud Network Models in Physics and Atmospheric Sciences
Cloud Microphysics
Description: Cloud microphysics models model the formation and interaction of cloud particles (e.g., water droplets, sugar crystals).
Objective: Understanding the growth and interaction of particles can help predict cloud conditions such as precipitation and cloud cover.
Large Eddy Simulation (LES)
Description: LES focuses on resolving large-scale turbulent structures in the atmosphere while modeling small, unresolved scales.
Objective: Information on wind and cloud activity, essential for accurate weather forecasting.
Cloud-Resolving Models (CRM)
Description: CRMs model individual cloud systems in great detail and describe the interactions between clouds and their environment.
Objective: Understand processes such as cloud formation, expansion, and dispersion at high resolution.
2. Cloud Network Models in Information and Networks
Scheduling and Resource Allocation
Description: Examples of work scheduling and resource allocation in cloud computing environments.
Purpose: To optimize the use of resources (e.g., CPU, memory) across multiple virtual machines.
Techniques: Algorithms for load balancing, priority planning, and resource aggregation.
Topology and Network Performance
Description: Models that simulate the structure and behavior of networks in cloud computing environments.
Techniques: Network traffic simulation, error detection, and mitigation strategies.
Service Level Agreements (SLA)
Description: Models that manage and monitor SLAs in cloud environments.
Objective: Ensure that service providers meet performance and availability expectations.
Methods: Monitoring and analysis tools to track adherence to SLAs and take corrective action as needed.
3. Cloud Network Models in Science and Technology
Monte Carlo Simulation
Description: Statistical models use random sampling to study the properties and behavior of materials.
Method: Involves generating a number of random configurations and analyzing their properties.
Molecular Dynamics (MD)
Description: Simulations of molecular and atomic interactions over time to study the behavior of materials.
Purpose: Analyzing dynamic properties such as viscosity, elasticity, and structural stability.
Applications: Used in material design, drug discovery, and understanding biological processes.
Permeability Theory
Description: Studies the movement and filtration of fluids through porous materials.
Objective: Understand properties such as material strength, permeability, and fluid flow.
Technique: Uses network models to simulate fluid flow in random pore networks.
4. Applications and Implications
Climate Science
Purpose: Cloud network models in climate studies help predict how changes in cloud cover and its characteristics will affect global warming and weather patterns.
Benefit: Improved understanding of feedback mechanisms in climate systems.
Disaster Management
Objective: Better weather forecasting and prediction of severe weather events will aid in preparedness and response.
Impact: Can reduce damage and improve safety by providing timely warnings and risk assessments.
Material Innovation
Objective: Simulation helps in the development of new materials with properties suitable for various application areas.